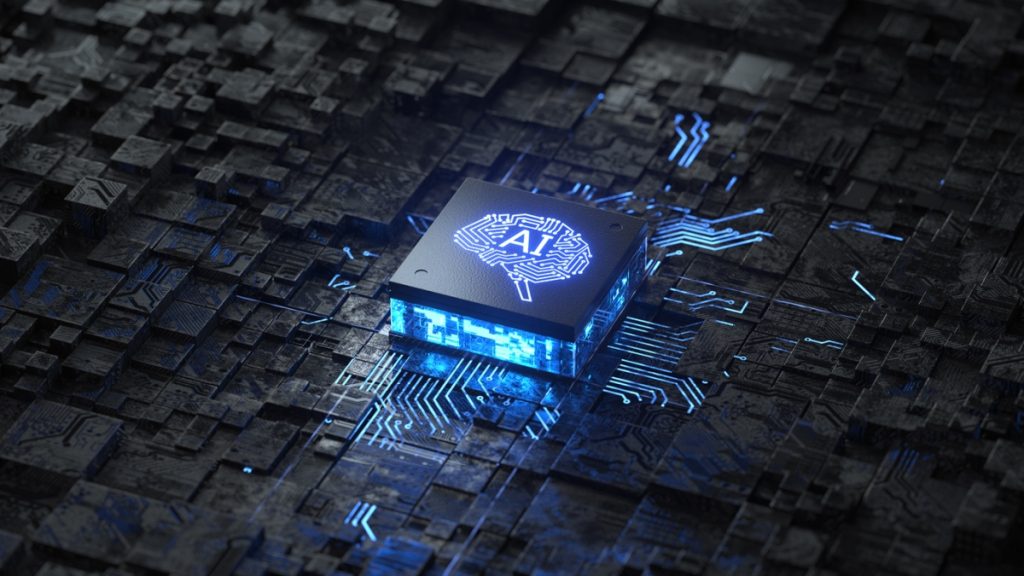
The secrets of the Google ranking algorithm are a mystery. Forget Colonel Sander’s blend of spices and the Coca-Cola recipe, the Google algorithm is the biggest trade secret on the planet. And Google is right to be secretive. The algorithm has helped to make Google one of the richest companies in the world.
But, occasionally, Google gives us mere mortals a little glimpse into what makes search tick. The latest peek behind the curtain came in the shape of a blog post titled “How AI powers great search results”.
This week we put Google’s post into a wider context of how AI in Google search has evolved. For instance, how do the various AI components of the algorithm work together? We will also let you know if there is anything you can do with this knowledge to improve your search strategy.
Let’s “search” for some answers.
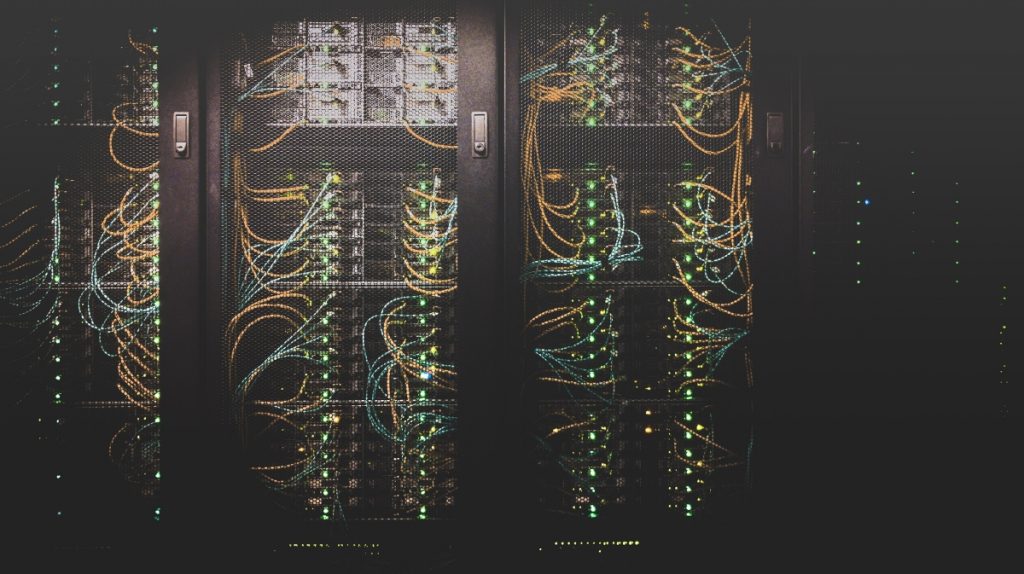
AI and SEO – The Different AI Algorithms Google Uses
It is important to note that the “Google Algorithm” is not one master algorithm. It consists of many (some say over 200) different algorithms that each look at different factors.
In the blog post Pandu Nayak from Google highlights four key AI algorithms that help to power Google search.
They are:
- RankBrain
- Neural Matching
- BERT
- MUM
Each of these algorithms tackles a different problem that users face in getting relevant results from a query. In fact almost all of Google’s recent patents in search have focused on information retrieval. Retrieving better quality information in different ways does seem to be Google’s overriding strategy in organic search.
So let’s look at each of these algorithms and what they actually do.
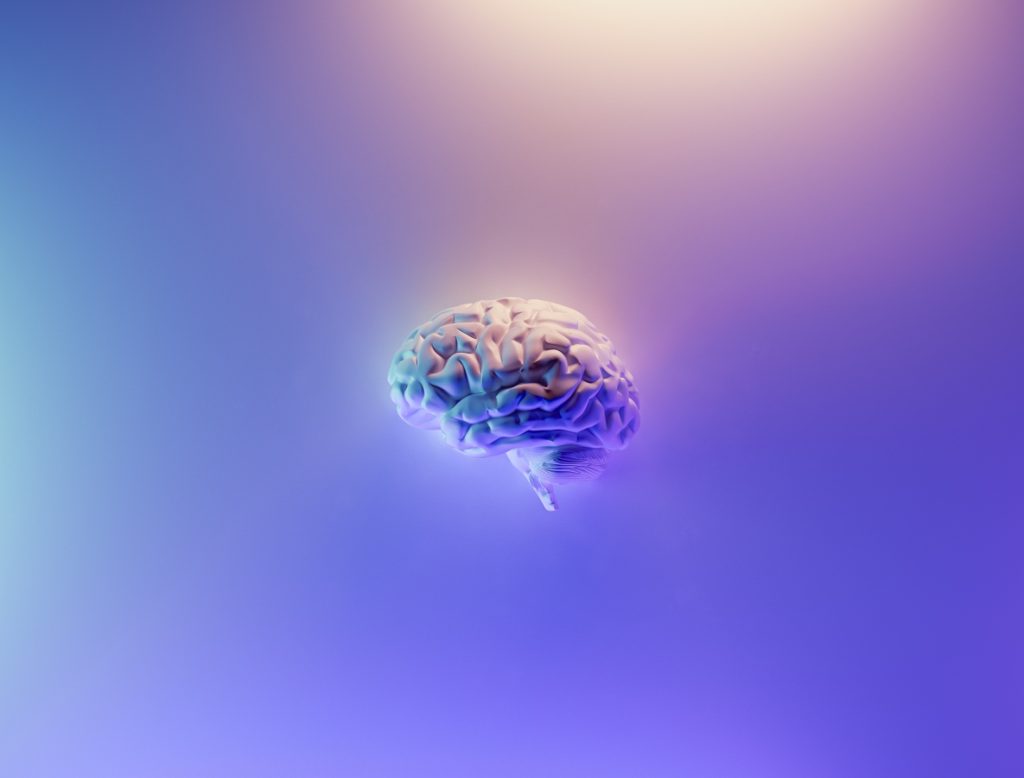
AI and SEO – RankBrain
Google confirmed the use of RankBrain in the Google Search algorithm back in 2015.
RankBrain was Google’s first AI system and, at the time, incredibly innovative. It’s core function was to interpret relationships between words and concepts. That might sound strange to a person, but computers are really not very good at understanding this. Put simply RankBRain tries to figure out what you actually mean when you are typing a query. So rather than just matching keywords, it turns your query into a concept and tries to find pages that are relevant to that concept.
So RankBrain helps Google to retrieve better quality information for users by understanding how words in a search query actually relate to real-world concepts.
But that’s only one part of the story. How does RankBrain know that the results it’s suggested are any good?
It does this by using user experience/behavior data.
RankBrain is still in use today and, more crucially, it helps to determine ranking for the top results of a user’s query. And RankBrain does this by looking at how users interact with the rankings it presents to them.
If users do not appear to be satisfied with the results they are getting, RankBrain changes them. It can also interact with other algorithms in search to increase or decrease their importance.
RankBrain can change search results on the fly, in nanoseconds, to present a better experience to users. But what user experience factors does it use?
User Experience Factors in RankBrain
RankBrain looks at the following user behaviors in Google Search:
- Click-through rate – Users clicking a result on organic search and going to a website
- Dwell Time – The amount of time that user spends on the website before returning to search
- Pogo-sticking – Where a user clicks on a result, immediately goes back to search and continues clicking results, which implies they are not getting the results they want
RankBrain is one of the most important parts of the Google Algorithm and something that businesses should be thinking about when implementing their organic search strategy (more on that later).
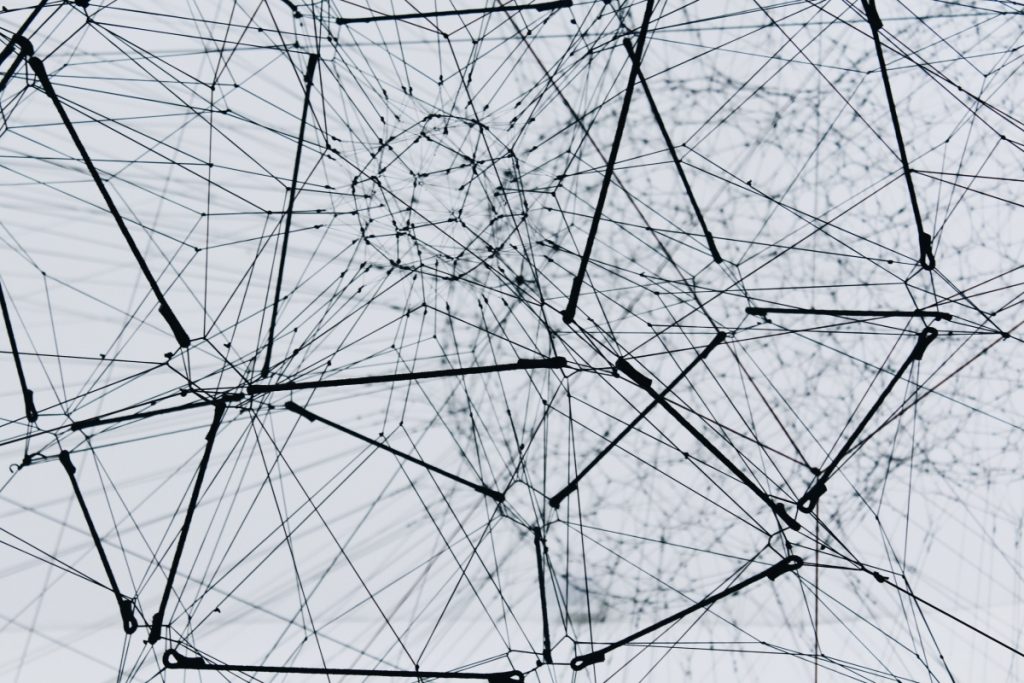
AI and SEO – Neural Matching
Neural Networks are one of the building blocks of many AI systems. In simple terms, Neural Networks are an advanced Machine Learning technique that help computers to learn with algorithms that are inspired by how the human brain works.
Google introduced Neural Matching into search back in 2018. Neural matching is largely used for information retrieval. It looks specifically at how search queries relate to pages.
Part of RankBrain’s job is turning your query into a concept. Neural Matching does a similar job but it looks at concepts and queries that are not very clear and then matches them to each other.
This is one of the ways that Google handles strangely phrased queries. Neural Matching looks at the whole query or page rather than just keywords to understand the broad underlying concept. It helps the Google Algorithm to actually decipher what the user wants when the query is not clear.
This better understanding of concepts, that is helped by Neural Matching, also helps to retrieve information from Google’s index. Google can find better, more relevant content because it can match that content to the concepts in the user’s query. As the sheer amount of content in Google’s index increases, Neural Matching is a vital part of how Google can find and retrieve the right information from its index.
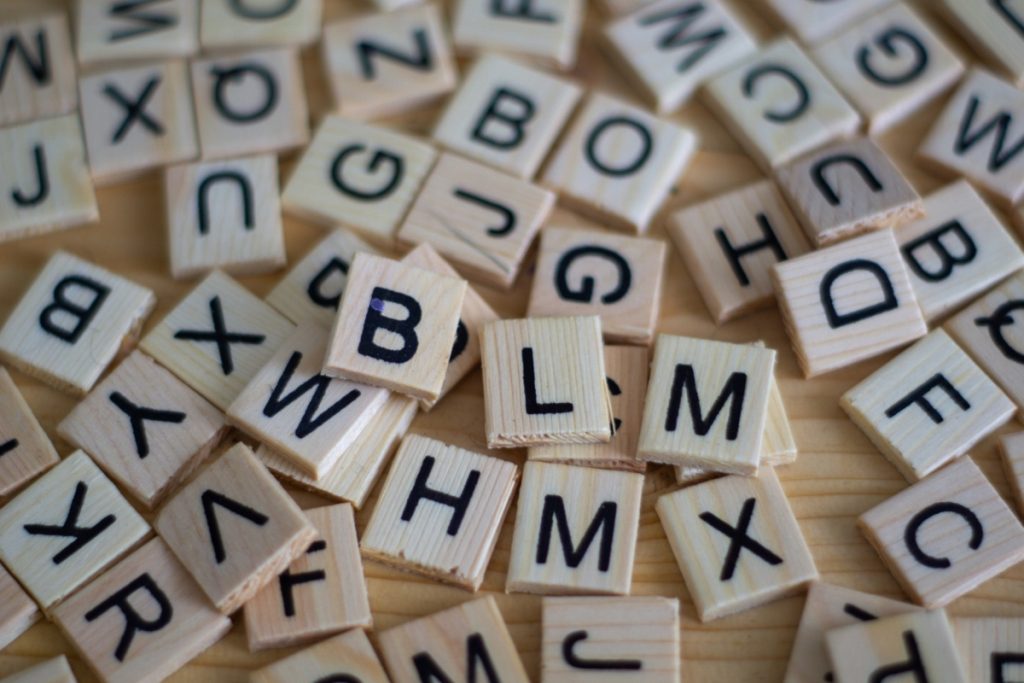
AI and SEO – BERT
Bidirectional Encoder Representations from Transformers, or BERT for short, is a neural network based technique for natural language processing (NLP). BERT was launched in Google Search in 2019.
BERT represented a big leap forward in how the Google algorithm understands language. It helped the Google algorithm to understand how combinations of words express different meanings and user intents.
Rather than looking around for content that matches words, BERT helped the algorithm to understand how a combination of words express a complex idea or concept. It can look at words in a sequence and how all the words relate to each other, which ensures that Google doesn’t drop words from a user query.
With BERT the results returned from queries became better as even the addition of the smallest word can change the intent of a query. Before BERT, those small words may have been ignored which would not return the type of results the user wanted.
BERT is now involved in almost every query Google receives in English. There is a multilingual BERT model too so it’s safe to assume BERT will be used by Google everywhere at some point in the future (if it isn’t already).
Like RankBrain and Neural Matching, BERT is directly involved in both retrieving relevant documents and ranking those documents to make sure the users get what they want. As the model can understand very complex language, BERT can rank documents very, very quickly.
BERT now plays a major role in the Google Search algorithm. It’s also used in what Google calls “legacy systems” and it’s likely used in Google Drive, Gmail and anything else that involves information retrieval.
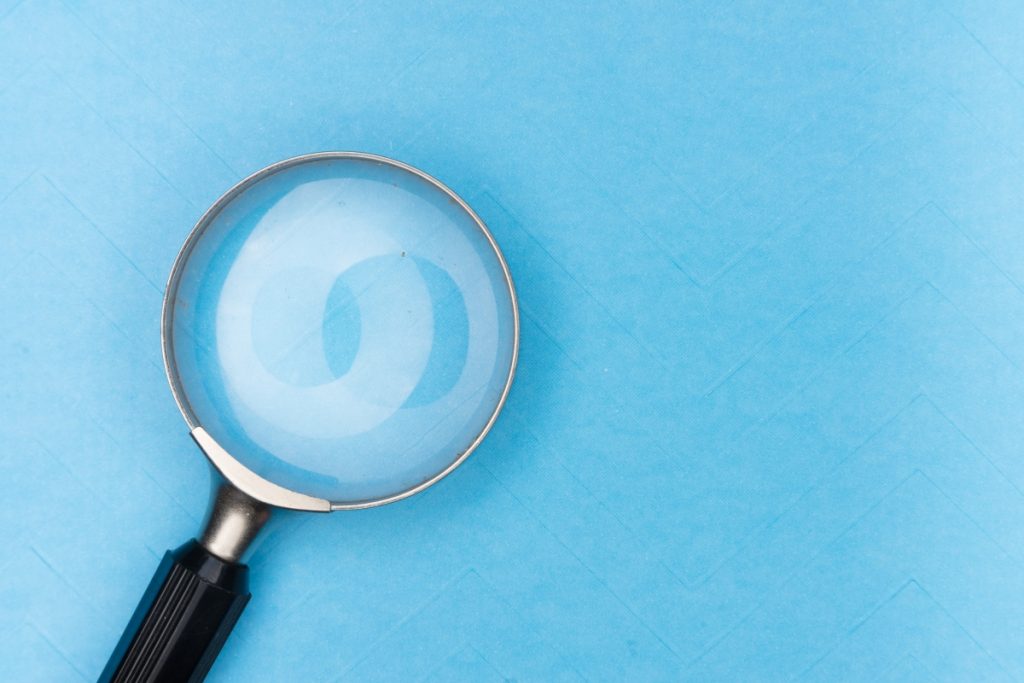
AI and SEO – MUM
Google MUM launched in May 2020 and it is Google’s latest AI milestone that has potentially huge implications.
We have written about Google MUM before, so we will not go into huge depth about it again here. But, put simply, it moves Google from language understanding to understanding information across many different mediums. MUM can be used on text, images and eventually it will be able to work on video.
Unlike RankBrain, Neural Matching and BERT, Google MUM is not currently used in the Google algorithm for ranking purposes or to improve the quality of search results. It’s uses so far have been specialized cases such as COVID-19 vaccine information, where it’s ability to quickly learn and transfer knowledge across the languages it is trained on decreased the time it took for Google to have relevant vaccine information in search in over 75 different languages.
MUM is part of the future of Google search and its use will only increase. But for now it’s not part of the Google Search algorithm for ranking and quality purposes.
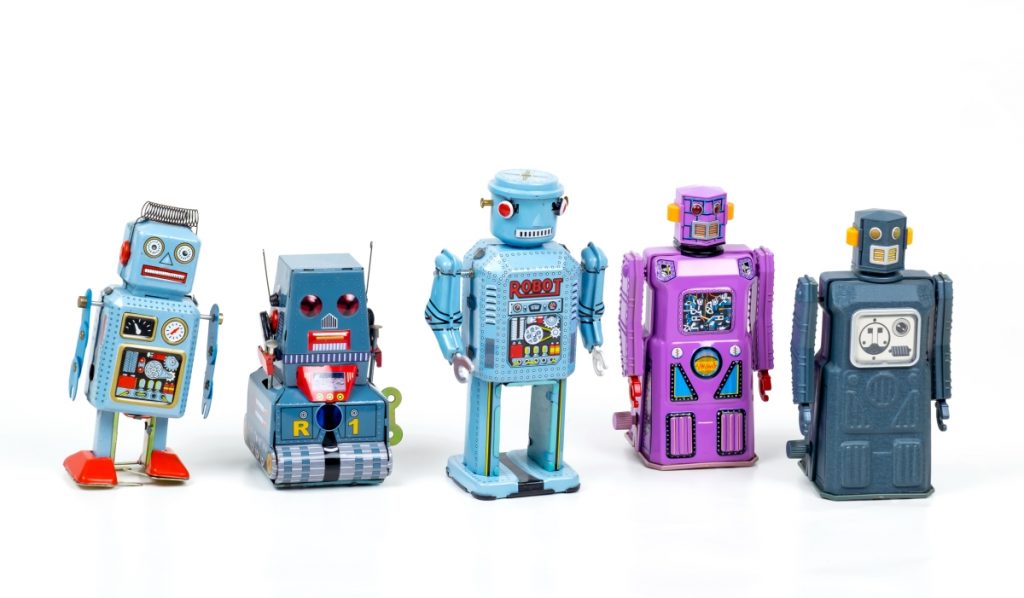
AI and SEO – How The Algorithms All Work Together
Google uses hundreds of algorithms and models to make search work. In fact, according to Google, old systems and models do not get shelved. New advances are used to improve older systems.
Each of the algorithms described above has a specific role and they are used at different times, in different combinations, to provide the best possible results to users. There is not “one thing” that you have to “optimize” for. There are hundreds of models and algorithmic combinations that are used to return search results.
Having said that, what should businesses consider when putting together a strategy for organic search? Not just for SEO in 2022, but even beyond that?
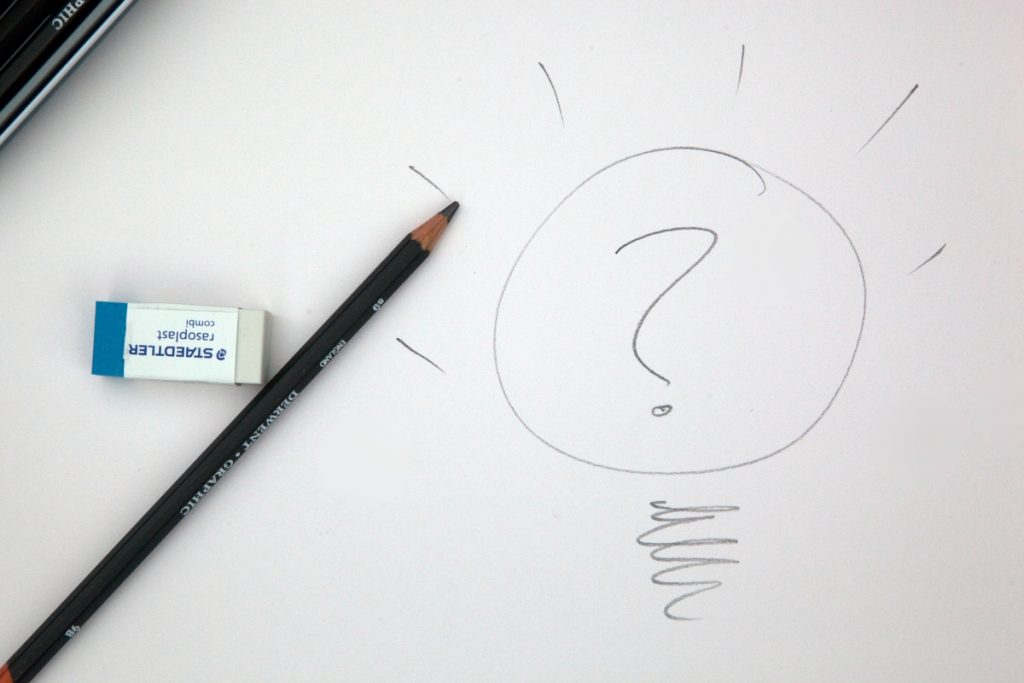
What This Means For Your Search Strategy
Most of these major AI advancements will have some effect on your search strategy, aside from Google MUM which is not yet used for ranking or quality purposes.
The biggest effect on search strategy comes in a few different areas. User experience factors, keyword research and then content creation.
RankBrain specifically touches all three of these areas. It looks at user behavior in search when the user interacts with your content. If the user does not appear to be satisfied, your content will fall down the rankings.
Keyword research used to involve a lot of work on “long tail” search, smaller terms with lower traffic terms that you could create specific pages to rank for as the landscape is less competitive. This is not as effective now as Google has moved more towards concepts than specific search strings, so those long tail keywords are less useful to your strategy.
This affects content creation. The content you create now needs to be more focused on broader concepts than specific keywords. Think about how the content you create is related to the industry you operate in. What do your users actually want? What information do they need? Try to create content on all your pages that gives users what they want. Intent personas can really help with this.
But for “checklist” purposes, the things any online business who wants to put together a solid SEO strategy that takes Google AI into account are:
- Optimize your titles and meta descriptions to improve your CTR from Google Search
- Optimize your content to improve dwell time (this involves creating more in-depth content, using headers to break up that content to make it digestible)
- Performing the above optimizations will minimize “pogo sticking”
- Make sure your site speed metrics are good (a slow site means users go back to search, which is “pogo sticking”)
- Create a topic structure for content, highlight relationships in your content with internal links
- Think about how your titles, descriptions and copy can fulfill user intent
- If you use product pages, make sure they have adequate information about the product
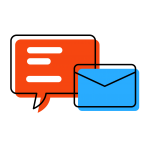
Talk to MAQE
If you need help adapting to the future of search and making sure your site can adapt to new changes from Google, talk to MAQE. We can offer you high-level strategic advice to make sure you can serve customers more effectively. Get in touch with us via [email protected].